Tasks are everywhere in our lives. We perform tasks to achieve goals (e.g., we exercise to stay healthy). Tasks make us more than reactively respond to environmental inputs and allow us to produce adaptive behaviors that are in line with internal goals. Tasks also lead to complex behaviors: Sometimes we perform tasks while preventing distractions from other tasks; sometimes we switch between tasks; sometimes we try to perform multiple tasks at the same time; sometimes we create/learn new tasks to achieve new goals. Although we all have a (rough) sense of what a task is conceptually, we know little about how the brain manages all the task information. Our research focuses on the fundamental questions about how task-set –– the collection of cognitive control demands required to perform a task –– is organized, learned, stored, retrieved and generalized to new tasks, contexts and experiences in young, aging and clinical populations. The methods used include lab- and web-based behavioral testing, functional neuroimaging, computational modeling and virtual reality technology. Below are several recent research directions:
The learning and memory of task-set
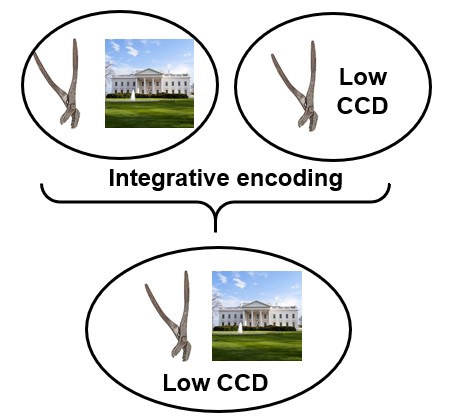
How do we retain knowledge of a vast variety of tasks (e.g., from cooking different dishes to solving math equations) and how do we learn new tasks quickly? The answer resides in how task-sets are organized and represented in the brain. We think that task-set is organized as cognitive maps such that similar tasks have similar mental representations (Lee, Hazeltine, & Jiang, in press, JEP:Gen; Bustos et al., in prep). Cognitive maps allow for organized representations to retain multiple tasks by compressing shared task information to increase storage capacity and to generalize to novel tasks. Hippocampal operations, such as pattern separation, pattern completion and reinstatement are also believed to support the learning and memory. of task-set.
Model-based cognitive control
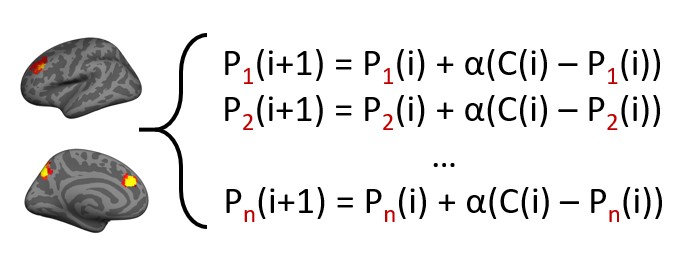
Cognitive control can be conceptualized as a set of modulatory parameters of information processing. We use computational modeling (e.g., Jiang, Heller, & Egner, 2014, Neurosci Biobehav Rev; Jiang, Beck, Heller, & Egner, 2015, Nat Commun) to simulate how the brain chooses and applies the set of parameters to implement cognitive control where and when it is needed (e.g., Jiang et al., 2020, Nat Commun; Jiang et al, 2020, J Neurosci; Muhle-Karbe, Jiang, & Egner, 2018, J Neurosci; Jiang, Wagner, & Egner, 2018, eLife). By combining generative models describing how model parameters give rise to behavior and Bayesian inferences that estimate the model parameters using empirical data, we can infer the mental states of human subjects and look for corresponding neural substrates. This approach allows us to propose quantitative hypotheses of cognitive control and to capture fine-grained learning and application processes of cognitive control.
How the white matter contributes to executive functions
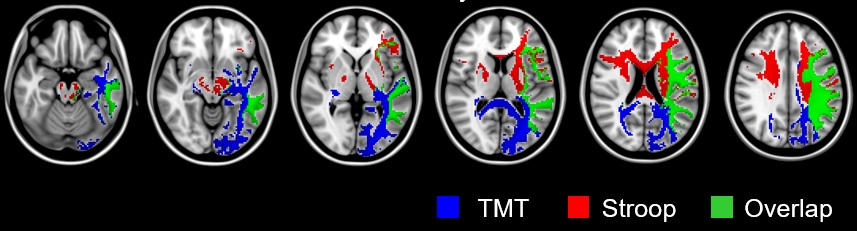
Compared to how much we know about the neural computations in the grey matter that support cognitive control and other executive functions (e.g., working memory), we understand very little about how the white matter contributes to cognitive control. Executive functions are implemented via coordinated neural activities across a wide range of cortical and subcortical areas. Thus, theoretically the white matter tracts connecting these areas must play an important role in the coordination process. However, the theory is hard to test, partly due to the technical difficulties in recording neural activities from the white matter. To address this issue, we leverage machine learning methods and the University of Iowa's large sample of patients with focal brain lesions and assess how white matter lesion is linked to post-lesion neuropsychological measures of executive functions (Jiang et al., 2023, Nature Communications).